Spatial Sampling and Statistical Inference related models are introduced here separately, which is helpful for users to understand the use of functions and the selection of sampling models.
Single-point Regional Estimation(SPA)
Under the condition that there is only one valid sample point for the survey object and the full coverage of the relevant variables with great correlation with the object can be obtained at the same time, the relevant variables are taken as covariates, and the unbiased estimation method of the population mean is carried out through the sample point. When the covariates are strongly correlated with the respondents, the model can achieve an unbiased estimate of the target population.
BShade model
In the case of biased samples, the existing data or covariates are used to calculate the correlation coefficient between each two samples, that is, the sample and the population, the sample mean and the population mean (the ratio of the estimated target), so as to realize the unbiased estimation of the biased sample to the population mean. When there is sufficient Historical Data or prior information to measure the ratio of each sample point to the target population (total or mean), and the covariance between two samples in the population can be calculated, the model can significantly improve the estimation accuracy.
Simple Random Sampling
A sample of n sample units is drawn from that population so that all possible combination of the n sampling units have an equal probability of being drawn. When there is no correlation and heterogeneity among the components of the population, the model can be selected; when there is correlation or heterogeneity among the components of the population, the sampling error of the model is large and the efficiency is low. Spatial correlation and spatial heterogeneity can be judged by Moran's I statistics and Geographic Detectorq , respectively.
System Random Sampling
Suppose the number of units in the population is N, the sample size is n, and the n units in the population are numbered 1, 2.. ,N。 First, a sample unit number is randomly selected, and then the other n-1 sample numbers are selected according to a preset order. This method is easier to operate than Simple Random Sampling.
Spatial Simple Random Sampling
Based on the Simple Random Sampling method, Spatial Autocorrelation is considered when calculating the sample size and Statistical Inference. The sample size required to achieve a given accuracy is less than using Simple Random Sampling. When the spatial distribution object has autocorrelation, but does not have spatial heterogeneity, the sampling efficiency of this method is better and the application is simple.
Stratum Random Sampling
Sampling is carried out independently in each layer, the total sample is composed of the samples of each layer, and the population parameters are estimated according to the summary of the parameters of the samples of each layer. Let the total sample size be n, and the sample sizes drawn from the L layers be N1, N2.. , nL, then N1 + N2 + nL = n. The sampling in each layer is carried out independently according to Simple Random Sampling, and the resulting sample is called stratified random sample. When the overall situation is complex, the difference between the components is large and the internal variation of the components is small, the advantages of this method are obvious. It is suitable for spatially distributed objects with heterogeneity and no autocorrelation.
Spatial Stratification Random Sampling
For the survey objects with spatial stratification heterogeneity, spatial classification can be carried out first, and then spatial stratified sampling method can be used for spatial distribution and Statistical Inference. The principle of layering is that the variance within the layer is small, the variance between the layers is large, and the points with relatively close attribute values are divided into the same layer and sampled independently in each layer. When the overall situation is complex, the difference between each layer is large, the internal difference is small and there is spatial correlation, the advantages of this method are obvious.
Sandwich Random Sampling
On the basis of spatial stratified sampling, a reporting unit layer (such as an administrative unit, a natural unit, or multiple units of interest to other users) is added, and a multi-unit and multi-reporting system can report at the same time. When the spatial differentiation of the respondents is obvious, the knowledge partition is close to the real differentiation of the respondents, the sample size is small, and the results need to be reported according to the designated multi-unit, the advantages of the model are obvious. It solves the problem of multi-reporting unit sampling with small sample size under the condition of population differentiation, and can realize multi-reporting unit reporting with less sample size.
The following figure shows the application of Sandwich Random Sampling:
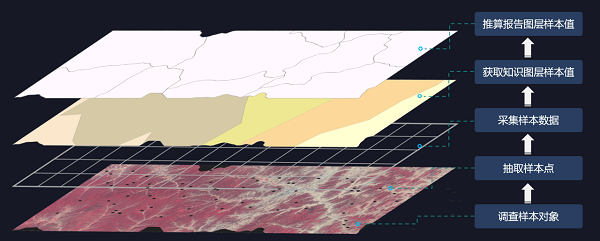
The following table summarizes the definition and characteristics of each model, which can be referred to and understood by users to facilitate the selection of appropriate models for Spatial Sampling and Statistical Inference.
Model Name | Description | Characteristic |
---|---|---|
Simple Random Sampling | The survey units are randomly selected from the population without any division of labor, classification, queuing, etc. | The probability of each sample unit being selected is equal, and each unit of the sample is completely independent, and there is no certain correlation and exclusion between them. Simple Random Sampling is the basis for all other sampling formats. |
System Random Sampling | The units of the population are arranged in a graphic or tabular form according to a certain mark and order, and then the sample units are taken at equal distances or intervals. | The extracted units are uniformly distributed in the population, and the number of samples extracted can be less than that of pure Random Sampling. Equidistant sampling can be queued either with a flag related to the survey item or with a flag unrelated to the survey item. |
Stratum Random Sampling | Firstly, the units of the population are divided into sub-populations (layers) according to certain characteristics, and then simple Random Sampling is carried out from each layer to form a group of samples. Stratification can improve the accuracy of the estimated value of the population index. It can divide a population with large internal variation into some layers (sub-populations) with smaller internal variation. | Because of the classification and stratification, the commonality of the samples in each type is increased, and it is easy to extract representative survey samples. |
Spatial Simple Random Sampling | On the basis of Simple Random Sampling, the sample units extracted from Spatial Autocorrelation are considered. When calculating the sampling precision, the spatial correlation between samples is considered. | The sample size is smaller than that of the simple random sample, the correlation is considered, the repeated sampling of the same kind of samples is reduced, the efficiency is improved, and the precision of the Calculate Result is higher. |
Spatial Stratification Random Sampling | On the basis of stratification, the sample units extracted from Spatial Autocorrelation are considered. When calculating the sampling precision, the spatial correlation between samples is considered. | Considering Spatial Autocorrelation, the sample points are less than those of stratified sampling, and the accuracy is improved. |
Sandwich Random Sampling | On the basis of spatial layering, combined with practical applications, the reporting unit layer (the reporting unit that users want to know) is added. | The traditional method is avoided, and the Calculate Result is directly calculated according to the report unit and the hierarchical relationship, which is convenient for the user, improves the accuracy, and reduces the sample size. |
Single-point Regional Estimation(SPA) | When the data features include both Spatial Autocorrelation and spatial differentiation, and the survey object has only one valid sample point, the sample point estimates the population mean unbiasedly. | Select the Single-point Regional Estimation (SPA) method when there is only one sample point |
BShade model | When the data features include both Spatial Autocorrelation and spatial differentiation, B-Shade model makes full use of the horizontal correlation of geographic space and the vertical correlation between the sample and the regional population, and is widely used in Statistical Inference with biased samples. | If there are no samples in some layers, the samples are biased, that is, the sample histogram is not equal to the overall histogram, and the BShade method with the ability to correct the bias is needed. |
Related topics
Single-point Regional Estimation